This paper introduces a cost-effective and low-power approach to unintentional fall detection. The authors utilize knowledge distillation-based LSTM models to improve accuracy. The focus is on analyzing time-series data from various sensors, enabling real-time detection for prompt and reliable identification of falls. The authors compare fall detection models based on different sensors and evaluate their accuracy rates and performance. Additionally, they apply knowledge distillation to enhance the precision of the models, resulting in refined configurations that consume less power. Overall, this proposed solution offers a promising pathway for the development of energy-efficient fall detection systems in the future.
Fall Detection on Offline Embedded and Low Power Devices using Knowledge Distillation Based Long Short-Term Memory
by instadatahelp | Aug 27, 2023 | AI Blogs
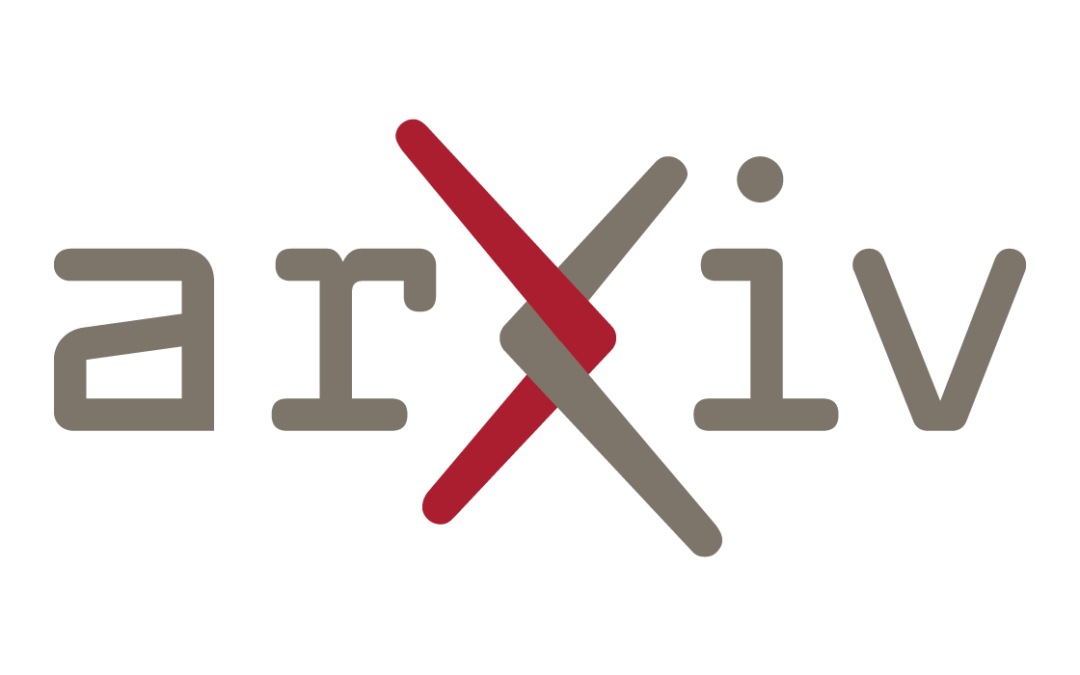