The Variational Autoencoder (VAE) is a widely used technique in drug discovery. However, it has been observed that VAE approaches struggle to accurately represent low-dimensional data in a higher-dimensional space. This issue has not been extensively studied in the context of drug discovery. In this study, we propose a multi-stage VAE approach to improve manifold recovery in drug discovery. We evaluate our approach using the ChEMBL dataset and demonstrate its ability to generate molecules with improved property statistics compared to existing methods that do not use property predictors in the training process. Additionally, we fine-tune our models on two smaller molecule datasets targeting different proteins. The results show that the multi-stage VAE generates a higher number of active molecules compared to the one-stage equivalent. We also compare our approach to methods that incorporate target metrics using learned property predictors and discuss the challenges associated with this approach.
Enhancing Molecule Properties through Multi-Stage VAE: An Objective-Agnostic Approach (arXiv:2308.13066v1 [cs.LG])
by instadatahelp | Aug 28, 2023 | AI Blogs
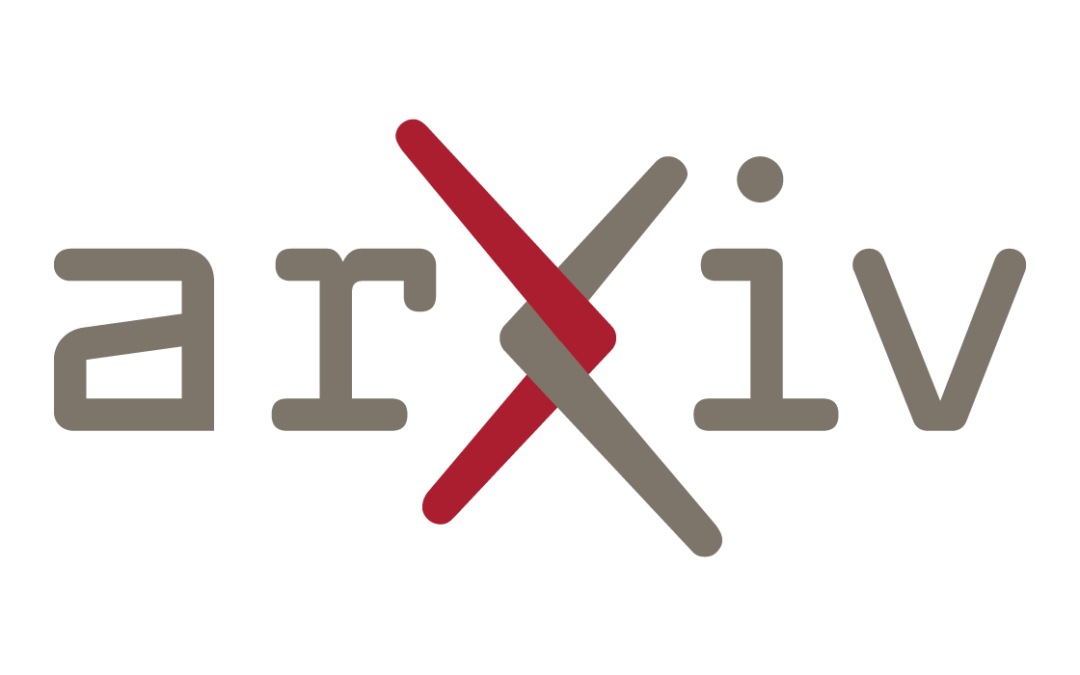