This research paper discusses the integration of the Internet of Things (IoT) ecosystem with Federated Learning (FL), a decentralized machine learning technique. FL is commonly used to collect and train machine learning models from various distributed data sources. The paper explores the challenges and solutions associated with implementing FL in resource-constrained IoT environments, specifically focusing on client and server levels. It addresses issues such as limited client resources, presence of heterogeneous client data, server capacity, and high communication costs. The effectiveness of these solutions in different scenarios is assessed, and the solutions are categorized based on their application location (IoT client or FL server). Additionally, the paper introduces new evaluation metrics for assessing solutions on resource-constrained IoT devices. Along with a comprehensive review of existing research and potential future directions, this paper aims to provide valuable insights and guidance for researchers in this field.
A Survey on Federated Learning in IoT: Considering Resource Constraints (arXiv:2308.13157v1 [cs.LG])
by instadatahelp | Aug 28, 2023 | AI Blogs
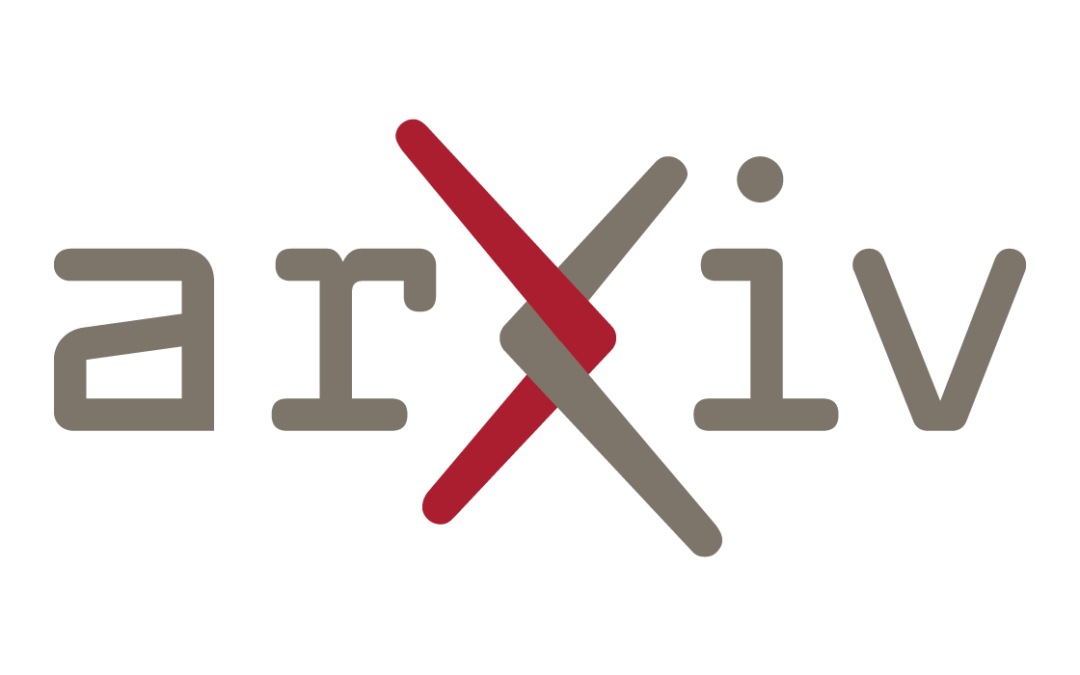