Our paper introduces Neural Adaptive Smoothing via Twisting (NAS-X), a novel approach for learning and inference in sequential latent variable models using reweighted wake-sleep (RWS). NAS-X is designed to handle both discrete and continuous latent variables and utilizes smoothing Sequential Monte Carlo (SMC) to accommodate a wider range of models compared to traditional RWS methods. Through experiments on discrete and continuous tasks, we demonstrate that NAS-X significantly surpasses previous variational and RWS-based methods in terms of inference accuracy and parameter recovery.
Neural Adaptive Smoothing via Twisting: Introducing NAS-X (arXiv:2308.14864v1 [cs.LG])
by instadatahelp | Aug 30, 2023 | AI Blogs
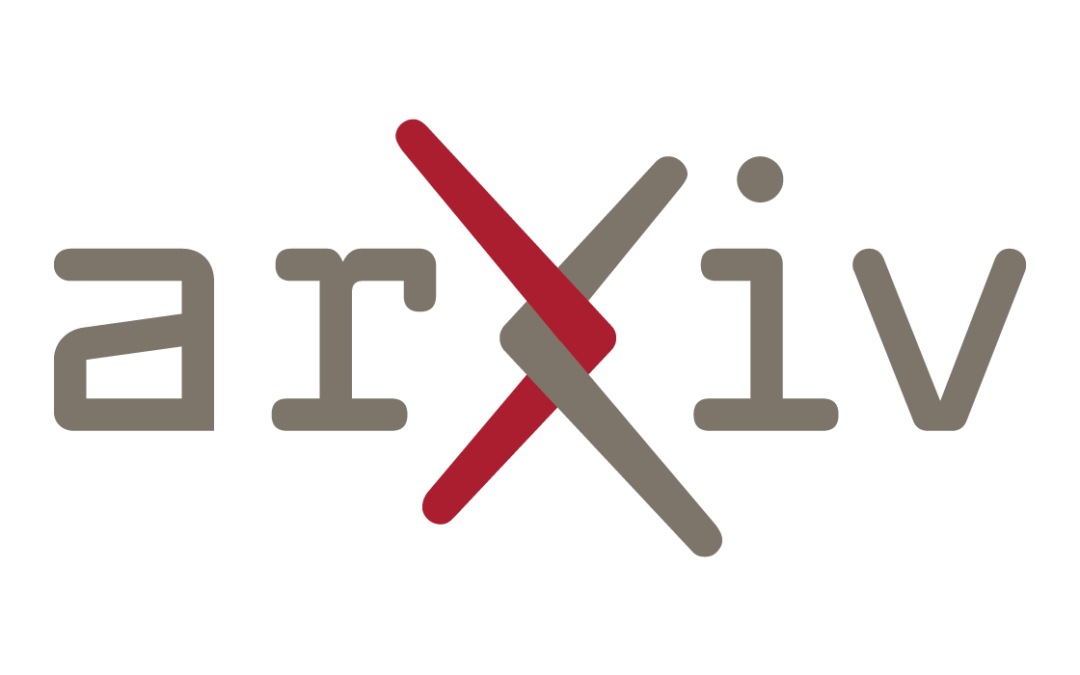