This paper presents a new method called Prox-SubGrad for solving nonconvex and nonsmooth optimization problems without the need for Lipschitz continuity conditions. The authors introduce several subgradient upper bounds and discuss their relationships. These upper bounding conditions are used to establish uniform recursive relations for the Moreau envelopes in weakly convex optimization. This uniform scheme simplifies and unifies the proof schemes for analyzing the convergence rate of Prox-SubGrad. Additionally, the paper proposes new stochastic subgradient upper bounding conditions and provides convergence and iteration complexity rates for the stochastic subgradient method (Sto-SubGrad) in solving non-Lipschitz and nonsmooth stochastic optimization problems. The authors show that, for weakly convex optimization problems without Lipschitz continuity, both the deterministic and stochastic subgradient methods achieve a convergence rate of $O(1/\sqrt{T})$ in terms of the square of the gradient of the Moreau envelope function. This convergence rate further improves to $O(1/T)$ if the uniform KL condition with exponent $1/2$ is also satisfied.
An Integrated Examination of Subgradient Methods in the Optimization of Composite Nonconvex, Nonsmooth, and Non-Lipschitz Functions.
by instadatahelp | Sep 1, 2023 | AI Blogs
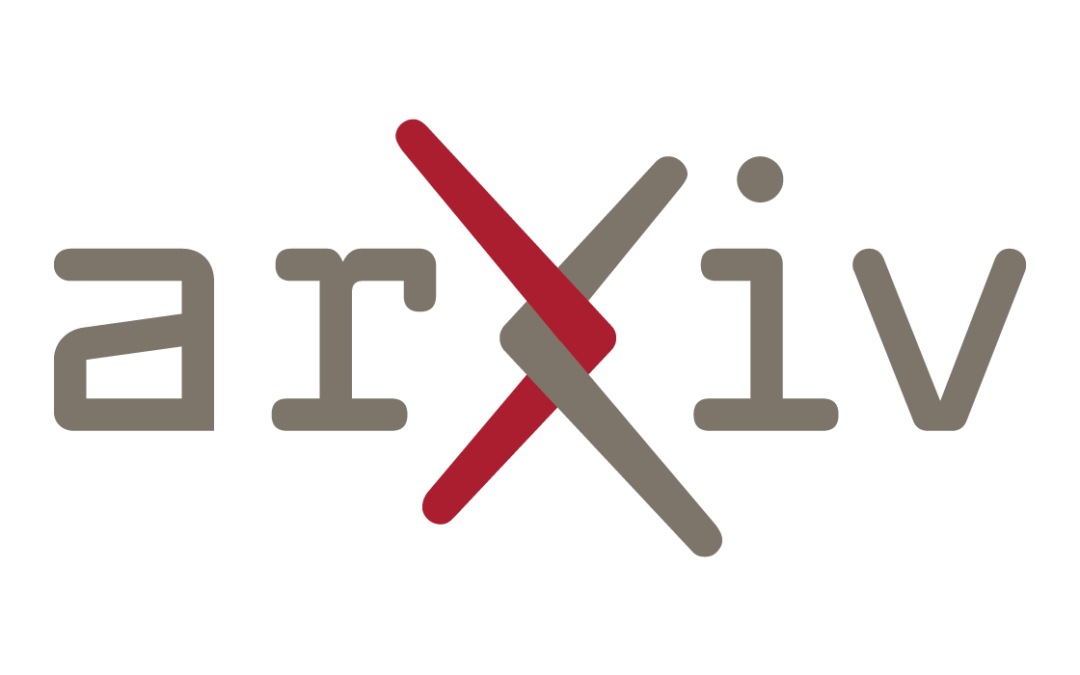