Ultrasound imaging is a crucial tool for diagnosing cervical lymph node lesions. However, the accuracy of these diagnoses heavily relies on the expertise of medical professionals, making the process prone to misdiagnoses. While deep learning has significantly improved the diagnoses of various ultrasound images, there is still a significant research gap when it comes to cervical lymph nodes. Our objective is to enhance the accuracy of diagnosing cervical lymph node lesions by utilizing a deep learning model. We began by collecting 3392 images that included normal lymph nodes, benign lymph node lesions, malignant primary lymph node lesions, and malignant metastatic lymph node lesions. Since ultrasound images are produced through sound waves reflecting and scattering across different bodily tissues, we proposed a Conv-FFT Block. This block combines convolutional operations with the fast Fourier transform to more effectively model the images. Based on this foundation, we developed a new architecture called US-SFNet. This architecture not only identifies differences in ultrasound images from the spatial domain but also accurately captures microstructural changes across various lesions in the frequency domain. To evaluate the potential of US-SFNet, we compared it to 12 popular architectures using five-fold cross-validation. The results demonstrate that US-SFNet is state-of-the-art and achieves an accuracy of 92.89%, precision of 90.46%, sensitivity of 89.95%, and specificity of 97.49%.
A Multi-Branch Network for Diagnosing Cervical Lymph Node Lesions in Ultrasound Images: US-SFNet in the Spatial-Frequency Domain (arXiv:2308.16738v1 [eess.IV])
by instadatahelp | Sep 3, 2023 | AI Blogs
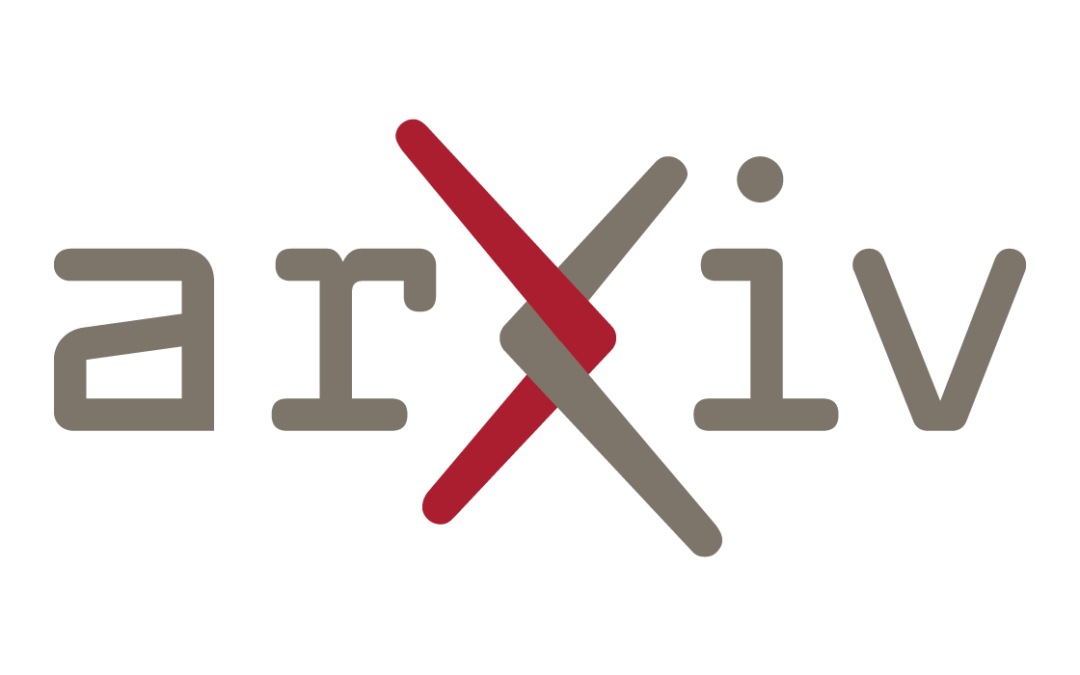