Predicting stock prices over a long-term period is crucial for various financial purposes such as forecasting volatility, pricing derivatives, hedging, and risk quantification. It is also important for institutional investors to have a liquidity horizon of several days to exit their risky assets without significantly impacting market prices. However, multi-step stock price prediction is challenging due to the stochastic nature of stock data. Existing solutions mainly focus on single-step, classification-based predictions and have limited representation expressiveness. The problem becomes increasingly difficult when considering target price sequences, which introduce stochastic noise and reduce generalizability during testing. To address these issues, we propose a combination of a deep hierarchical variational-autoencoder (VAE) and diffusion probabilistic techniques for seq2seq stock prediction through a stochastic generative process. The hierarchical VAE enables us to learn complex and low-level latent variables for stock prediction, while the diffusion probabilistic model trains the predictor to handle stock price stochasticity by gradually introducing random noise to the stock data. Our Diffusion-VAE (D-Va) model outperforms state-of-the-art solutions in terms of prediction accuracy and variance. Additionally, the multi-step outputs allow us to form a stock portfolio over the prediction length. We demonstrate the effectiveness of our model outputs in the portfolio investment task using the Sharpe ratio metric and emphasize the importance of addressing different types of prediction uncertainties.
Tackling Stochasticity in Multi-Step Regression Stock Price Prediction through Diffusion Variational Autoencoder
by instadatahelp | Sep 4, 2023 | AI Blogs
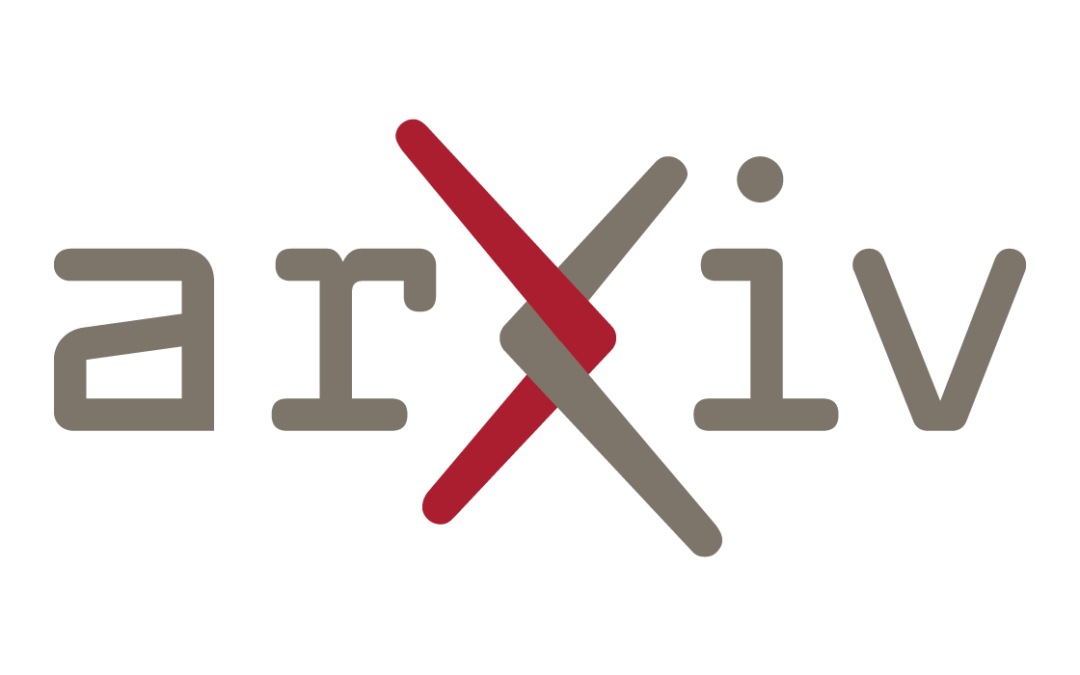