We present a novel approach called the Independent Component Laplace Process (ICLP) mechanism for releasing differentially private functional summaries. Unlike previous methods that assume finite-dimensional data trajectories, our approach treats the functional summaries as infinite-dimensional objects and perturbs them with ICLP noise. This relaxation of assumptions allows for higher utility compared to classical finite-dimensional subspace embedding techniques. We validate the feasibility of our mechanism in various function spaces and consider several statistical estimation problems. By slightly oversmoothing the summary, we show that the privacy cost is asymptotically negligible and does not dominate the statistical error. Furthermore, we conduct numerical experiments on both synthetic and real datasets to demonstrate the effectiveness of our proposed mechanism.
Differentially Private Functional Summaries using the Independent Component Laplace Process
by instadatahelp | Sep 4, 2023 | AI Blogs
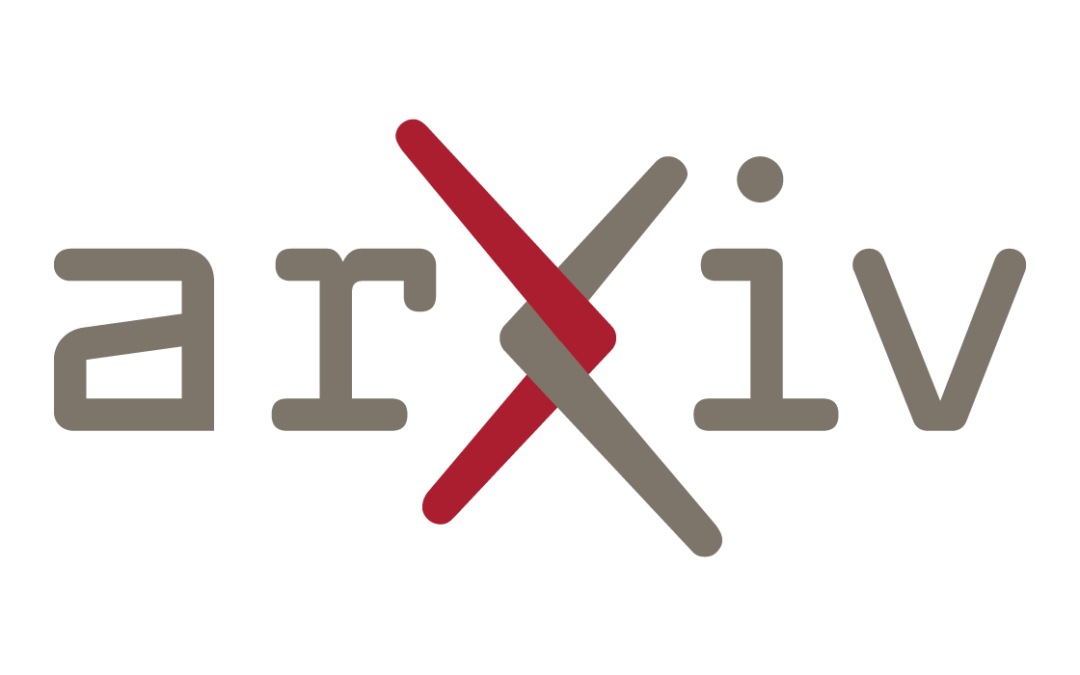