The fast movement of rubidium-82 ($^{82}$Rb) and the wide variation in cross-frame distribution in dynamic cardiac positron emission tomography (PET) pose significant challenges for correcting motion between frames, especially in the early frames where traditional intensity-based image registration techniques are not effective. Instead, a promising approach is to use generative methods to handle changes in tracer distribution and assist existing registration methods. To enhance frame-wise registration and parametric quantification, we propose a Temporally and Anatomically Informed Generative Adversarial Network (TAI-GAN) that transforms the early frames into the late reference frame using a one-to-one mapping. Our method employs a feature-wise linear modulation layer that encodes channel-wise parameters generated from temporal tracer kinetics information, along with rough cardiac segmentations that include local shifts as anatomical information. We tested our method on a clinical dataset of $^{82}$Rb PET and observed that TAI-GAN produces converted early frames with high image quality, comparable to the real reference frames. Following TAI-GAN conversion, motion estimation accuracy and clinical myocardial blood flow (MBF) quantification improved compared to using the original frames. Our code can be accessed at https://github.com/gxq1998/TAI-GAN.
TAI-GAN: A GAN with Temporal and Anatomical Information for Correcting Dynamic Cardiac PET Motion in Early-to-Late Frame Conversion
by instadatahelp | Aug 27, 2023 | AI Blogs
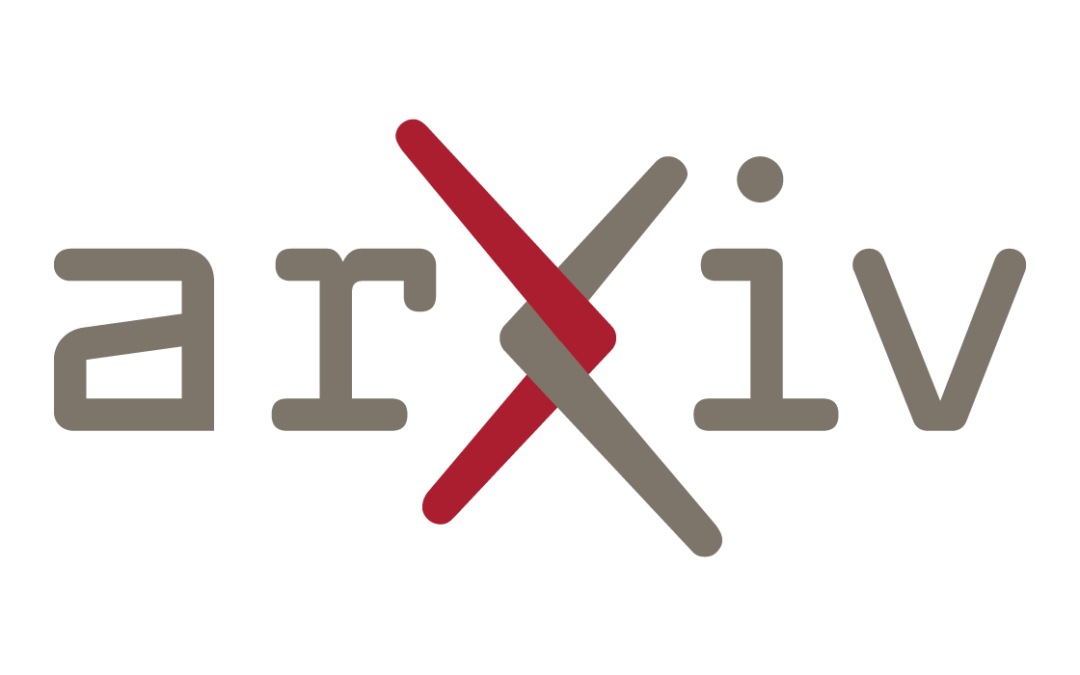